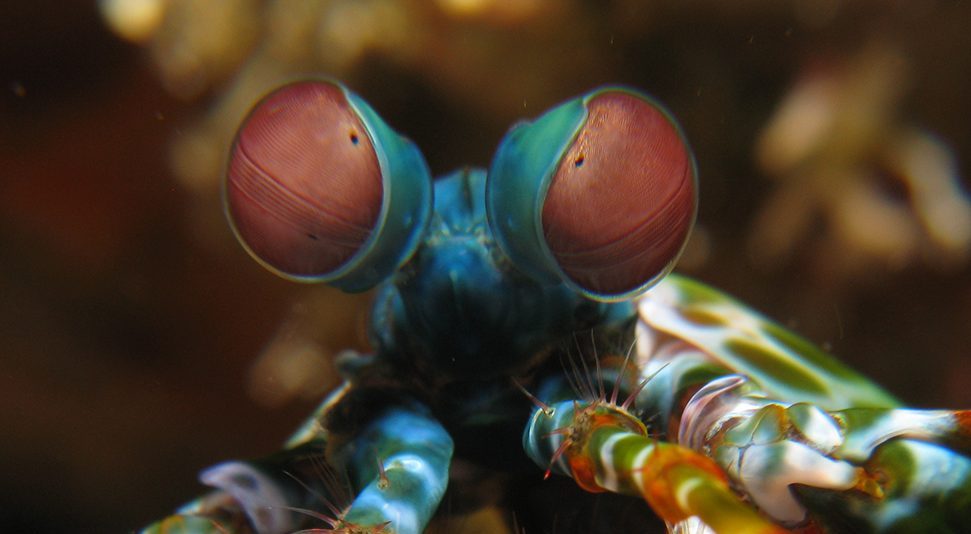
Navigating the future of underwater geolocalization
Beneath the water’s surface lays a hidden world: one that cannot be perceived by the human eye. When viewed through a special camera, however, rich polarization patterns are unveiled. These patterns can be used as an alternative approach to geolocation- the process of determining the geographic position of an object.
By: Amber Rose
University of Illinois Urbana-Champaign researchers have developed a novel method for underwater geolocalization using deep neural networks that have been trained on 10 million polarization-sensitive images collected from locations around the world. This new study, led by electrical and computer engineering professor Viktor Gruev, along with computer science professor David Forsyth, enables underwater geolocalization using only optical data while providing a tool for tethered-free underwater navigation.
These findings were recently published in the journal eLight.
“We are showing for the first time, you can geolocate yourself, or a camera, in a number of different conditions, whether in open ocean waters, clear waters or low visibility waters, at day, at night, or at depth,” says Gruev. “Once you have a sense of where you are, then you can start exploring and use that information to have a better understanding of the underwater world or even how animals navigate.”
Gruev explains that one of the main challenges with underwater navigation and geolocalization is that GPS signals cannot penetrate water- they bounce off the surface. “We are blind in terms of GPS signals underwater. We need to use different means and different technology for geolocating underwater.”
The current standard for geolocalization is using acoustic information, mainly obtained by sonar technology. This works by deploying many little sonar beacons which send signals that are triangulated to locate an object underwater. The issue, however, is that sonar only works in a small, defined area, while also being limited by its accuracy.
Another method currently utilized is using submersibles that are tethered to a larger vessel above the surface that has a GPS signal. Although the submersible can maneuver a little bit, it is ultimately limited by the movement of the vessel.
“It is an incredibly challenging problem to have a free-moving, underwater vehicle. The way we solve this problem is by developing specialized cameras and machine learning algorithms. By combining those, we can actually figure out the sun’s location and this is where polarization imaging comes into play.”
–Victor Gruev
Light waves from the sun move in all directions-it is unpolarized. When those waves pass through a filter, like the water’s surface, they are forced to move in only one direction-the light has been polarized. Polarization patterns are the result of light’s transmission from the air to the water and scattering by water molecules and other particles. The patterns underwater change throughout the day, and they depend on the location of both the observer and the sun. By analyzing these patterns alongside accurate date and time information, it is possible to then determine location.
The team collected ~10 million images with an underwater camera and an omnidirectional lens capable of recording the polarization patterns from four sites: a freshwater lake in Champaign, IL (visibility around 0.3 m), coastal sea waters in Florida Key, FL (visibility around 0.5-3 m), sea water in the bay of Tampa, FL (visibility around 0.5 m), and a freshwater lake in Ohrid, North Macedonia (visibility exceeding 10 m). Images were taken in a variety of conditions (clear vs murky waters), depths, and times of the day- even at night when underwater light intensity is significantly weaker.
“We think of life as dull if we can’t see anything, if we cannot see the hands in front of us. But if we can see polarization properties of light, we can geolocate, even in muddy waters. And actually, life is pretty rich in terms of polarization,” Gruev says.
These images were used to train a neural network-a method of artificial intelligence to learn and improve accuracy over time. “The way we did this was to collect 10 million images of the sun from underwater,” Forsyth explains. “Each image was tagged with where it was taken, and the elevation of the sun. Those images were then passed into a learning system and the system was adjusted until it gave a precise location.” Using these machine learning techniques has helped improve location accuracy to 40-50 km, with the possibility of improving accuracy even further.
This technology presents new opportunities for people and robots to navigate underwater. Oceans account for over 70% of Earth’s surface area, yet very little is known about it. Data we do know about these bodies of water comes from monitoring via satellites 20-30 miles above the surface. In situautonomous sampling robots could provide more precise monitoring of water properties such as water temperature, salinity, oxygen levels and other related parameters.
The recent OceanGate Titan submersible search and rescue efforts have highlighted the need for accurate geolocation abilities. In order to locate the submersible at any possible depth, efforts were split into two distinct regions, near the ocean surface and near the seafloor, due to the limitations of current technology. Deep water efforts are significantly more challenging than near-surface operations, which have more technological options, and rely mainly on sonar. Not only is sonar unreliable over a large area, but it also often creates echoes that conceal an object’s precise location. Gruev says, “This polarization imaging technology will enable smaller autonomous robots to roam around the first 200-300 meters where light penetrates into the water and where our technology works very well and can help during search and rescue missions.”
“It is difficult to understand just how big the oceans are, how much water there is, how far away from anything you can be, and how hard it is to find anything out there. The biggest technological problem, up until the early 19thcentury, was simply knowing where you were at sea. And it remains really, really difficult.”
–David Forsyth
Viktor Gruev is also an affiliate of Beckman Institute for Advanced Science and Technology, the Department of Bioengineering and the Carle Illinois College of Medicine at UIUC.
Other contributors to this work include Xiaoyang Bai (Department of Electrical and Computer Engineering at UIUC), Zuodong Liang (Department of Electrical and Computer Engineering at UIUC), Zhongmin Zhu (Department of Electrical and Computer Engineering at UIUC) and Alexander Schwing (Department of Electrical and Computer Engineering and Department of Computer Science at UIUC).
This research was funded by the Office of Naval Research and U.S. Air Force Office of Scientific Research.
“Polarization-Based Underwater Geolocalization with Deep Learning”
You May Also Like

Seeing the unseen: How butterflies can help scientists detect cancer
May 27, 2015